Retail isn’t broken – markdowns are.
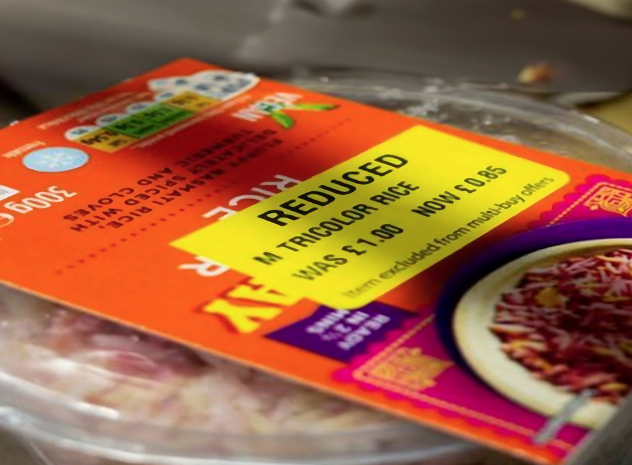
There has been a feverish activity around markdown optimization in the last few years. Originating in the early 1970s and evolving ever since, the reputation of markdowns transcended from being “inappropriate” to the “way of doing business.” If performed correctly, they offer significant revenue engines, generating profit from products that would otherwise be thrown away. However, since the first ever markdown was applied, many great changes took place all across the retail landscape – from the stores themselves, volume of retail, supply chains, and supporting retail technologies, to the preferences and needs of consumers. To help you to better navigate these emerging intricacies, we will review three key strategies for applying markdowns, in increasing order of sophistication and performance
“Gut-Feel” Markdowns: Instead of using advanced analytics, these markdown strategies often rely on arbitrary price decisions driven by a set of parameters and not supported by any in-depth analysis. Such “guesstimation” approaches are usually dependent on an employee’s individual abilities, far from optimal and typically result in price reductions that are too steep, thus diminishing revenues for the retailer. The timing and markdown amount cannot be optimal if they are solely based on intuition rather than data.
Rule-Based Markdowns: A more advanced approach to apply markdowns in retail. Most online retailers adjust their pricing using this strategy, but it is often also applied in “more advanced” brick-and-mortar stores. Following this practice, prices are updated according to static formulas and parameters, which usually include its remaining shelf life, the category involved, or time of day. Thus, prices can be changed more often and rapidly, to respond to the real-time needs and behavior of both the market and consumers.
There are two main disadvantages to rule-based markdowns:
- They comprise only limited parameters, which set forth the rules.
- They lack flexibility and hence leave little to no room for optimization over time.
Many retailers that regularly apply rule-based markdowns tend to abandon these before fully realizing revenue and profit potential.
Dynamic Markdowns: This data-driven markdown strategy relies on artificial intelligence (AI), particularly machine learning (ML) algorithms, to employ “educated” and dynamic markdowns based on an up-to-date shelf status of inventory. At Wasteless, we offer our retailers dynamic markdown solutions that may be applied on both the SKU level and per expiration date. As part of the Wasteless approach, markdowns are optimized and adjusted by employing a ML-framework that can process a large and flexible set of variable arrays such as sell-in, sell-out, shelf status, expiration dates, competitor pricing, demand, time, traffic, location, conversion rates, sales’ goals, and other internal and external factors – involved in markdown efforts. Naturally, important data may not always be available or may often be too “noisy”. The Wasteless solution establishes a robust algorithmic framework for addressing such data discrepancies and still maintain optimized markdowns.
The main advantage of dynamic markdown algorithms is that the consumer’s behavior is not expected to match any pre-set pattern – there is a solid, non-linear, and non-consistent relationship between price and demand. Therefore, it can be challenging to suggest a markdown without exploring the relation of demand and pricing for the specific product and category. This is the pitfall that many rule-based markdown patterns fall into.
Advantages of dynamic markdown optimization in supermarkets:
- "Dynamic markdowns will improve the percentage of products sold from the shelf by focusing on which product to sell first."
- Supermarkets increase profits, avoiding costly markdown practices that don’t hold up over time.
- Markdown optimization is a proven way to avoid food waste and its staggering costs.
- Markdown optimization reacts almost immediately to consumption patterns.
- Markdown optimization dynamically optimizes the relationship between price and expiration dates, keeping supermarket shelves consistently fresher. Fresher shelves = higher quality products = happier customers.
- Markdowns, if operationally feasible, should be applied if and when required, potentially multiple times in throughout a product’s shelf life.
Dynamic Markdowns Vs. Rule-Based Markdowns:
|
Rule-Based Markdowns |
Dynamic Markdowns |
Markdown Initiator |
|
|
Markdown Timing |
|
|
Markdown Size |
|
|
Operational Efficiency |
|
|
Financial Performance |
|
|
Focus on Improvement |
|
|